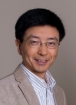
Professor Xiaoyi Jiang
University of Münster, Germany
3D depth images have turned out to be a key
information source for solving a large number of challenging applications.
Substantial advances have been demonstrated to acquire, process, analyze, and
interpret depth images. Meanwhile, depth data plays a vital role in a variety
of application areas including human gesture and pose estimation, object
recognition, biometrics, cultural heritage applications, and 3DTV (e.g. Depth
Image Based Rendering). Through the recent development in consumer depth
cameras, in particular the low-cost Kinect, a new era of depth data analysis
emerged. Affordable depth cameras are changing the landscape of computer vision
and related research fields, with profound impact far beyond consumer
electronics. The objective of this tutorial is to present an overview of techniques
for depth acquisition, processing, analysis and interpretation, and
applications.
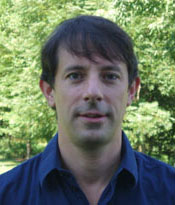
Professor Daniel P. Lopresti
Lehigh University, USA
Tutorial:
Challenging Pattern Recognition Problems from the Field of Document Analysis
The field of document
analysis has developed over the past 50 years, starting with what was once considered
to be a simple task – recognizing clean, typeset text – to a wide range of much
more difficult problems driven by attempts to build systems that replicate – or
even exceed – human levels of perception and understanding. As a target application for pattern
recognition research, documents provide a uniquely rich set of challenges. In this tutorial, we will survey fundamental
problems from the field of document analysis, examine pattern recognition
techniques that have proven to be effective, and identify some open problems
that are still the subject of active research today.

Oklahoma State University, USA
Tutorial:Using
Neural Networks for Pattern Recognition, Function Approximation, Clustering and
Prediction
This tutorial will begin with an introduction to the
basic types of neural networks. This will be followed by a discussion of how
neural networks can be used to solve practical problems in pattern recognition,
function approximation, clustering and prediction. Case studies of each type of
application will be presented in some detail, and students will have the
opportunity for hands-on experiences in training and testing a variety of
neural networks.
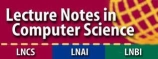
